What is AI?
A look at technology's most exciting, yet most misunderstood, field of research
From C-3PO and R2D2 to the Replicants in Blade Runner, artificial intelligence (AI) has always been a fixture in classic Hollywood movies. On the silver screen, AI is usually presented as robots (Chappie) or virtual assistants (Samantha in Her) meaning to question the definition of humanity. Their role often is to show the horrors of a dystopian world in a far-away future.
However, not many of us realise that, even in present-day reality, it is hard to go by a single day without coming into contact with AI-powered tech.
Expected to be the most disruptive technology ever, or at least since the Industrial Revolution, AI is everywhere: in offices, laboratories, and likely your home. It is there to help you order Friday night takeaway (if you use apps such as UberEats or Deliveroo), vacuum your flat, or wake you up in the morning to your local radio station (“Alexa, wake me up at 7am to BBC Radio London”). AI is also out there detecting and mitigating cyber attacks for cyber security as well as alerting the military of any incoming threats.
Over the last few years, it has proven that it is extremely adaptable to almost any given sector and it is often more reliable than humans themselves. That is why AI has earned itself the reputation of a job-stealer and most predictions show that it will continue to fill even more positions as time goes on. However, one man's loss is another man's gain, and the UK's booming AI sector is attracting talent from all over the world, with companies such as Oracle expanding their AI base in England as opportunities increase and development thrives.
Yet, although we hear the term quite a lot, AI is, in fact, a largely misunderstood and misquoted field of research. It's a term that's often used interchangeably with machine or deep learning (ML & DL), and because of this, the nuances of AI are generally ignored.
Examples of AI
AI is considered an umbrella term for a range of different technologies. As such, how AI is deployed varies significantly.
The automotive industry, in particular, has seen dramatic change over the past few years as a result of AI. The idea of creating an autonomous vehicle capable of responding in real time to dangers on the road is something that most major manufacturers are now experimenting with.
For most people, their first encounter with a form of AI will be through smart home devices like the Amazon Echo or Google Home. These smart assistants rely on accurate voice recognition to relay data from the internet, whether that's in the form of answers to questions, queries about the weather, or playing songs from a music streaming platform. Although data analysis of this kind is relatively surface level, devices such as these give us a glimpse of the everyday role that AI could one day play.
However, AI is not without its controversy. Public bodies and organisations across the world are becoming concerned about the development of the technology, particularly when it comes to eliminating potential bias.
Algorithms are already being used to help inform highly sensitive decisions, such as the length of a custodial sentence based on criminal history, or whether to hire someone based on their CV. This is despite growing concern that a lack of diversity in the development of the tech is leading to algorithms either ignoring or favouring certain demographics. As a result, the development of 'ethical AI' is now a focus of governments both in the UK and across Europe.
However, in order to fully understand the complexities of AI, it's important to breakdown the various dimensions of the term.
Weak AI vs strong AI
We encounter the simplest form of AI in everyday consumer products. Known as 'weak AI', these machines are designed to be extremely intelligent at performing a certain task. An example of this is Apple's Siri, which is designed to appear very intelligent but actually uses the internet as its information source. The virtual assistant can participate in conversations, but is limited to doing so in a restrictive, predefined manner that can lead to inaccurate results.
On the other hand, in its most complex form, AI may theoretically have all the cognitive functions a human possesses, such as the ability to learn, predict, reason and perceive situational cues. This 'strong AI' can be perceived as the ultimate goal, but humans have yet to create anything deemed to be a fully independent AI.
Currently, the most compelling work is situated in the middle of these two types of AI. The idea is to use human reasoning as a guide, but not necessarily replicate it entirely. For example, IBM's supercomputer Watson can sift through thousands of datasets to make evidence-based conclusions.
Applied vs general
Perhaps a more useful way of defining AI is to look at how it is deployed.
Applied, or 'narrow', AI, refers to machines built for specific tasks. This has been the most successful application of the technology within an industry, allowing systems to make recommendations based on past behaviour, ingesting huge quantities of data to make more accurate predictions or suggestions. In this way, they can learn to perform medical diagnoses, recognise images, and even trade stocks and shares. Despite this narrow form of AI being brilliant in its own field, it isn't designed to perform day-to-day decision-making.
General AI remains the realm of science fiction. Instead of being trained on a specific type of data to perform one task very well, like applied (or narrow) AI, general AI would see a machine able to perform any task a human can. This would involve, for instance, it being able to learn a lesson from one type of situation and apply that lesson to an entirely new situation.
While general AI is generating a lot of excitement and research, it's still a long way off - perhaps thankfully, because this is the type of AI sci-fi writers discuss when talking about the singularity - a moment when powerful AI will rise up and subjugate humanity.
Machine learning & deep learning
While general AI may attract the most public attention, it's the field of applied AI that has had the greatest success and biggest effect on the industry. Given the focused nature of applied AI, systems have been developed that not only replicate human thought processes, but are also capable of learning from the data they process - known widely as 'machine learning'.
An example of this is image recognition, which is increasingly becoming an AI-led field. A system may be designed to manipulate pre-scripted routines that analyse shapes, colours and objects in a picture, scanning millions of images in order to teach itself how to correctly identify an image.
However, as this process developed it quickly became clear that machine learning relied far too much on human prompting and created wide margins of error if an image was blurry or ambiguous.
Deep learning is arguably the most powerful from of AI algorithm development and machine learning. In basic terms, deep learning effectively involved the creation of an artificial neural network, which is essentially a computerised take on the way mammal brains work.
The human brain for example is made up of neurons that are connected together with synapses in a massive network. This network will take in information, say what a person is viewing, and dissect with nuggets of data flowing to each neuron which works to figure out what it is viewing, say if part of an image contains a certain object or colour.
An artificial neural network does this only with the use of nodes rather than neurons, which dissect information perform a calculation and assign a value to how likely they feel it is they are viewing a certain colour or shape.
The deep learning part comes from the layers that artificial neural networks are made up of, as unlike the brain, the nodes are not all connected together. As such, in a deep learning neural network the idea is that once one layer finishes analysing the data being processed, it's then passed down to the next layer where it can be re-analysed using additional contextual information.
For example, in the case of an AI system designed to combat bank fraud, a first layer may analyse basic information such as the value of a recent transaction, while the second layer may then add location data to inform the analysis.
After data has passed through each layer the artificial neural network comes up with an answer to say, "is there a dog in this picture". If the answer it serves is correct then the network is correctly configured to at least attempt to spot dogs in pictures.
If not, then that data is then sent back through the network in a process called backpropagation whereby the network readjusts the values each nose has given to the data segment it looked at until is effectively comes up with the best possible answer; it's very difficult for a deep learning neural network to come up with a definitively correct answer so it seeks the most likely answer.
In the case of Google's AlphaGo, the system that defeated a champion Go player in 2016, the deep learning neural network is comprised of hundreds of layers, each providing additional contextual information. While machine learning is a type of AI, there are differences between the two terms - read more about them here.
Are there risks?
As with any technology, AI presents risks as well as opportunities. These fall largely into two categories: existential and economic.
On the economic side, the more advanced AI gets the more roles it will be able to fulfil that would otherwise have been performed by humans. For businesses at first blush at least this seems an excellent investment: while the initial outlay for an advanced AI system may be high, and there will be ongoing costs in terms of hosting and maintenance, it will likely cost less than the combined salaries of the people it's replacing, particularly when benefits, such as pensions, and taxes are taken into account.
While manufacturing and agriculture have been at the sharp end of this revolution, as they always have been in the past, other industries including law, education and journalism are now also at increasing risk of automation.
This becomes a particular problem if more jobs are removed from the economy than there are new ones to replace them, which gives rise to the spectre of growing unemployment. This, in turn, could drive down wages and reduce average disposable income, leading to fewer people being able to buy products and an ever slowing economy.
Various mitigations have been proposed for this problem, such as a four-day working week or universal basic income. Some people believe that such concerns are unwarranted, anyway, as previously unthought-of jobs will be created for humans and for AI systems.
The existential risks of AI are less pressing but potentially more serious.
These range from intelligent malware that can adapt on the fly to thwart cyber defences, to more sci-fi style ideas like Skynet from the Terminator series.
AI-powered malware used as a cyber weapon could devastate nation states in a targeted attack, causing long-term problems for that country not just at an administrative or infrastructure level, but also for residents trying to go about their day-to-day lives. Additionally, if there are any errors in coding or deployment, the potential exists for the creators to lose control of the malware, which could then turn against anyone and everyone including them.
In Terminator, the AI system Skynet gains self-awareness, decides (rightly or wrongly) that humans pose an existential threat to it, and so preempts our attempts to destroy it by destroying us with our own nuclear weapons. Thankfully, the possibility of a self-aware system is a long way away, assuming it's possible to create such a thing at all, so for now, we can put the idea in a box marked "James Cameron et al".
For the latest AI industry news, head to our hub.
Get the ITPro. daily newsletter
Receive our latest news, industry updates, featured resources and more. Sign up today to receive our FREE report on AI cyber crime & security - newly updated for 2023.
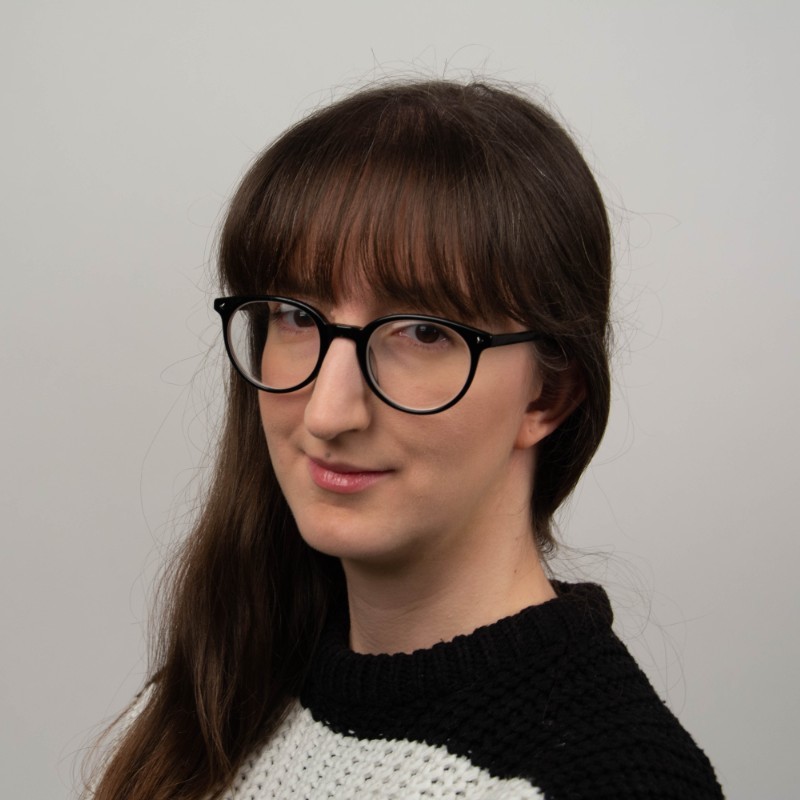
Jane McCallion is ITPro's deputy editor, specializing in cloud computing, cyber security, data centers and enterprise IT infrastructure. Before becoming Deputy Editor, she held the role of Features Editor, managing a pool of freelance and internal writers, while continuing to specialise in enterprise IT infrastructure, and business strategy.
Prior to joining ITPro, Jane was a freelance business journalist writing as both Jane McCallion and Jane Bordenave for titles such as European CEO, World Finance, and Business Excellence Magazine.